A Little Bit About Me
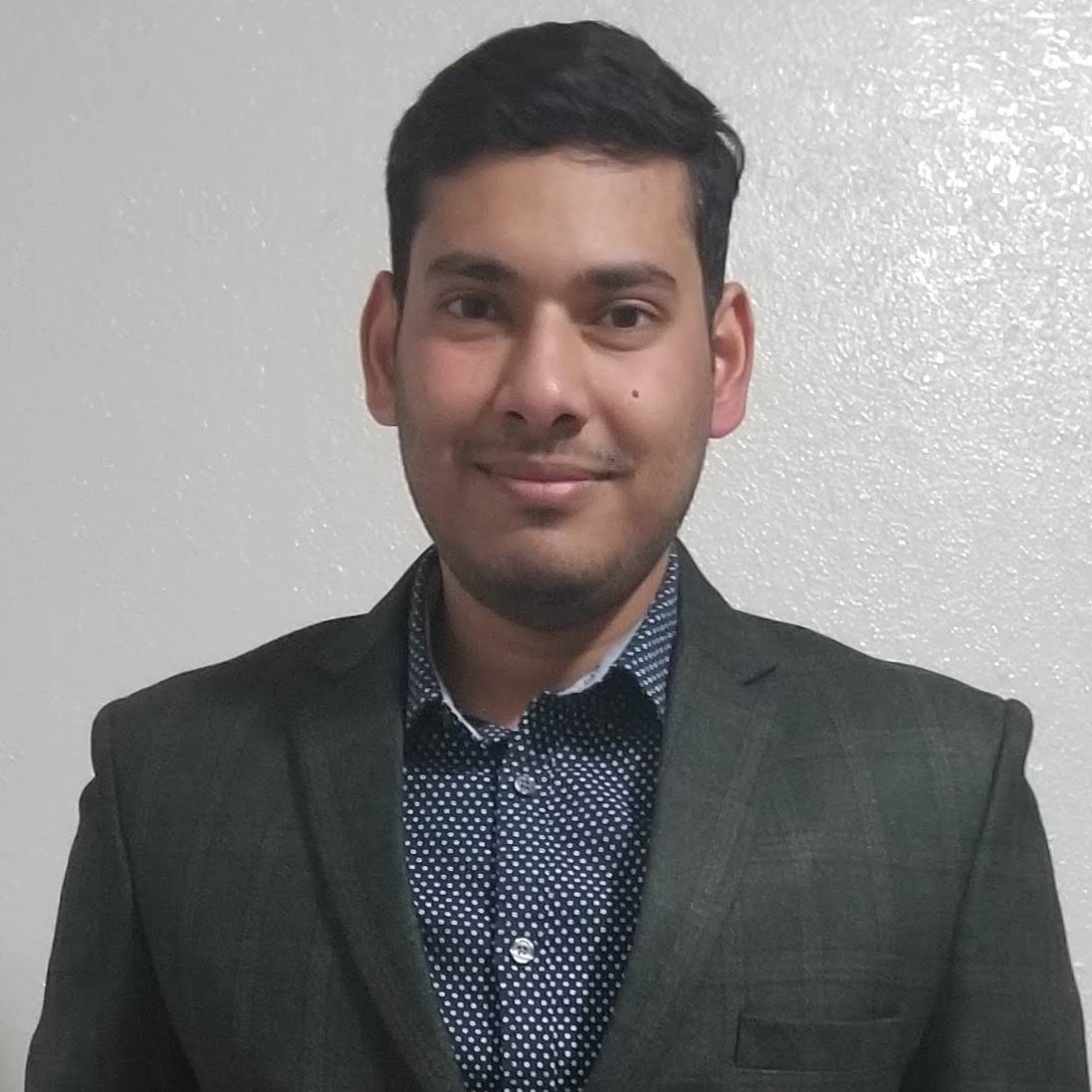
Greetings! My name is Rahul Sharma. I am an Applied Scientist at Amazon within the Artificial General Intelligence organization, dedicated to advancing responsible AI for cutting-edge multimodal large language models. I have earned my Ph.D. from Ming Hsieh Institute of Electrical and Computer Engineering, University of Southern California where I worked with Prof. Shrikanth Narayanan at the interesection of technology and human behavior understanding.
My research interest lies in multimodal signal processing, more inclined towards the visual signal (Computer Vision), to understand human actions and behavior in multimedia content. Furthermore, I am keenly interested in semi-supervised systems and the notion of weeker-than-full supervision.
Immersed in the captivating field of multimodal technologies, my academic journey has been devoted to unraveling their profound impact on understanding human behavior. During my time at USC, I dedicated my efforts to crafting innovative strategies for detecting active speakers in long-form media videos, pushing the boundaries of Computational Media Intelligence. Prior to this, I honed my skills at the prestigious Indian Institute of Technology, Kanpur, earning both my Bachelors' and Masters' degrees in Electrical Engineering. My master's thesis delved into the fascinating world of public speaking videos, where I developed a computational framework to quantify a speaker's performance. This transformative experience was guided by the expertise of Dr. Tanya Guha and Dr. Gaurav Sharma.
Check out my résumé!
Active Speaker Localization
Weakly supervised setup
An objective understanding of media depictions, such as about inclusive portrayals of how much someone is heard and seen on screen in film and television, requires the machines to discern automatically who, when, how and where someone is talking. Media content is rich in multiple modalities such as visuals and audio which can be used to learn speaker activity in videos. In this work, we present visual representations that have implicit information about when someone is talking and where. We propose a crossmodal neural network for audio speech event detection using the visual frames. We use the learned representations for two downstream tasks: i) audio-visual voice activity detection ii) active speaker localization in video frames. We present a state-of-the-art audio-visual voice activity detection system and demonstrate that the learned embeddings can effectively localize to active speakers in the visual frames.
Visual activity based ASD (ICIP'19) Poster ICIP'19
Vocal Tract Articulatory Contour Detection in Real-Time Magnetic Resonance Images
Due to its ability to visualize and measure the dynamics of vocal tract shaping during speech production, real-time magnetic resonance imaging (rtMRI) has emerged as one of the prominent research tools. The ability to track different articulators such as the tongue, lips, velum, and the pharynx is a crucial step toward automating further scientific and clinical analysis. Recently, various researchers have addressed the problem of detecting articulatory boundaries, but those are primarily limited to static-image based methods. In this work, we propose to use information from temporal dynamics together with the spatial structure to detect the articulatory boundaries in rtMRI videos. We train a convolutional LSTM network to detect and label the articulatory contours. We compare the produced contours against reference labels generated by iteratively fitting a manually created subject-specific template. We observe that the proposed method outperforms solely image-based methods, especially for the difficult-to-track articulators involved in airway constriction formation during speech.
Video Analysis for Autism prescreening
Ongoing
An effort to automatically understand the key characteristics of ASD kids’ behavior as with respect to TD kids using the videos of home and clinical sessions. The system involves classifying the humans present in the scene into child and interlocutor. Further it involves computing the features representing the child-interlocutor dynamics. To quantify the the child-interlocutor interaction, we compute the physical proximity between them and the gaze directions of their view and study their dynamics over time along with an additional speaker diarization information.
Eyetracking analysis for Cortical Visual Impairment
Ongoing
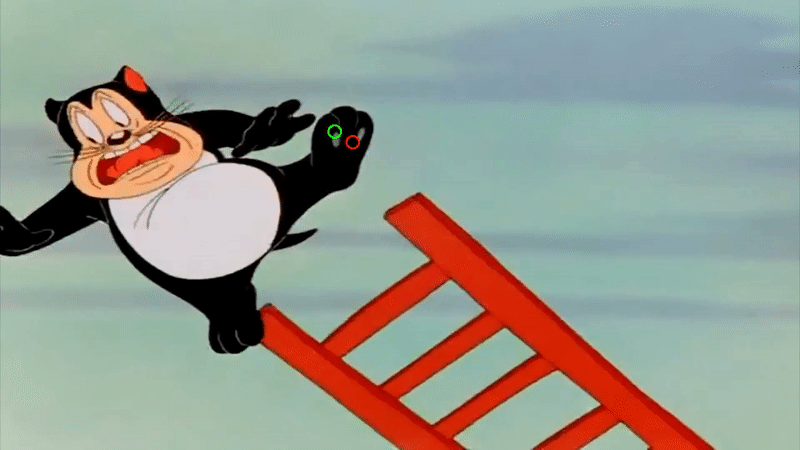
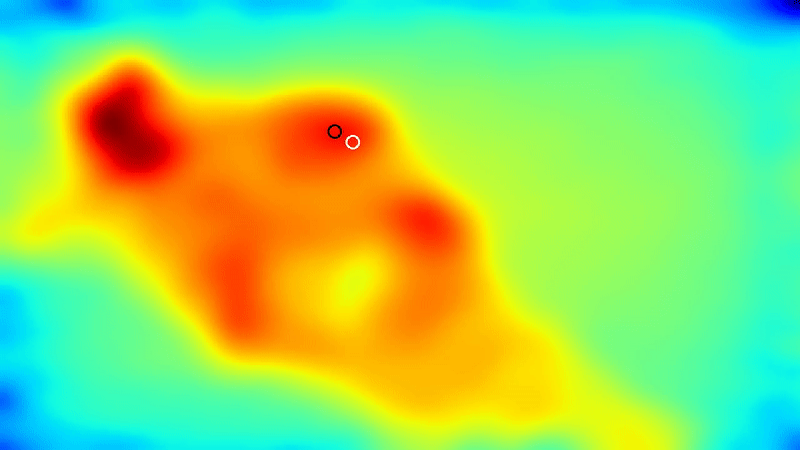
In this work we analyze the eyetracking data from the kids to study the key characteristicsin the visual patterns demostrted by the kids having Cortical Visual Impairment against control subjects. We compute the slaiency maps over the presented stimuli to generate a representation of the eyetracks.
Analyzing Visual Behavior in Public Speaking
We investigate the importance of human centered visual cues for predicting the popularity of a public lecture. We construct a large database of more than 1800 TED talk videos and leverage the corresponding (online) viewers' ratings from YouTube for a measure of popularity of the TED talks. Visual cues related to facial and physical appearance, facial expressions, and pose variations are learned using convolutional neural networks (CNN) connected to an attention-based long short-term memory (LSTM) network to predict the video popularity. The proposed overall network is end-to-end-trainable, and achieves state-of-the-art prediction accuracy indicating that the visual cues alone contain highly predictive information about the popularity of a talk. We also demonstrate qualitatively that the network learns a human-like attention mechanism, which is particularly useful for interpretability, i.e. how attention varies with time, and across different visual cues as a function of their relative importance.
Crowd Flow Segmentation in Videos
This work proposes a trajectory clustering-based approach for segmenting flow patterns in high density crowd videos. The goal is to produce a pixel-wise segmentation of a video sequence (static camera), where each segment corresponds to a different motion pattern. Unlike previous studies that use only motion vectors, we extract full trajectories so as to capture the complete temporal evolution of each region (block) in a video sequence. The extracted trajectories are dense, complex and often overlapping. A novel clustering algorithm is developed to group these trajectories that takes into account the information about the trajectories’ shape, location, and the density of trajectory patterns in a spatial neighborhood. Once the trajectories are clustered, final motion segments are obtained by grouping of the resulting trajectory clusters on the basis of their area of overlap, and average flow direction. The proposed method is validated on a set of crowd videos that are commonly used in this field. On comparison with several state-of-the-art techniques, our method achieves better overall accuracy